The Big Data Landscape looks like in 2016:
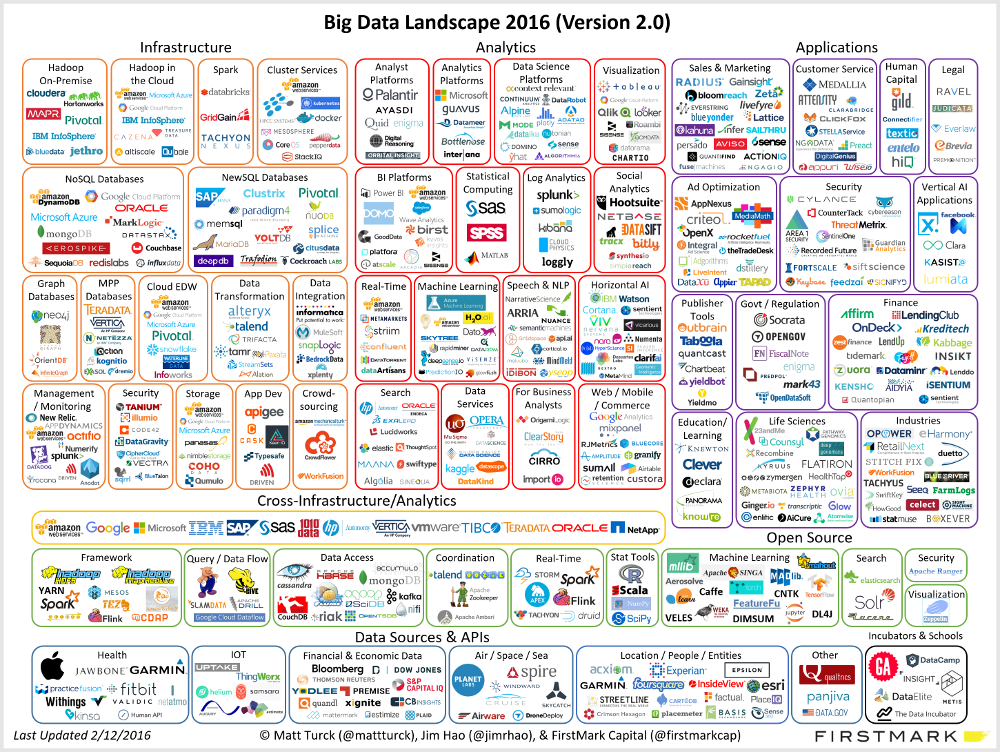
Obviously, that’s a lot of companies, and many others were not included in the chart, deliberately or not (scroll to the bottom of the post for a few notes on methodology).
In terms of fundamental trend, the action (meaning innovation, launch of new products and companies) has been gradually moving left to right, from the infrastructure layer (essentially the world of developers/engineers) to the analytics layer (the world of data scientists and analysts) to the application layer (the world of business users and consumers) where “Big Data native applications” have been emerging rapidly – following more or less the pattern we expected.
Big Data infrastructure: Still Plenty of Innovation
It’s now been a decade since Google’s papers on MapReduce and BigTable led Doug Cutting and Mike Cafarella to create Hadoop, so the infrastructure layer of Big Data has had the most time to mature and some key problems there have now been solved.
However, the infrastructure space continues to thrive with innovation, in large part through considerable open source activity.
2015 was without a doubt the year of Apache Spark, an open source framework leveraging in-memory processing, which was starting to get a lot of buzz when we published the previous version of our landscape. Since then, Spark has been embraced by a variety of players, from IBM to Cloudera, giving it considerable credibility. Spark is meaningful because it effectively addresses some of the key issues that were slowing down the adoption of Hadoop: it is much faster (benchmarks have shown Spark is 10 to 100 times faster than Hadoop’s MapReduce), easier to program, and lends itself well to machine learning. (For more on Spark, see our fireside chat at our Data Driven NYC monthly event with Ion Stoica, one of the key Spark pioneers and CEO of Spark in the cloud company Databricks, here).
Other exciting frameworks continue to emerge and gain momentum, such as Flink, Ignite, Samza, Kudu, etc. Some thought leaders think the emergence of Mesos (a framework to “program against your datacenter like it’s a single pool of resources”) dispenses for the need for Hadoop altogether (watch a great talk on the topic by Stefan Groschupf, CEO of Datameer and learn more about Mesos by watching Tobi Knaupf of Mesosphere).
Even in the world of databases, that seemed to have seen more emerging players than the market could possibly sustain, plenty of exciting things are happening, from the maturation of graph databases (watch Emil Eifrem, CEO Neo4j), the launch of specialized databases (watch Paul Dix, founder of time series database InfluxDB) to the emergence of CockroachDB, a database inspired by Google Spanner, billed as offering the best of both the SQL and NoSQL worlds (watch Spencer Kimball, CEO of Cockroach Labs). Data warehouses are evolving as well (watch Bob Muglia, CEO of cloud data warehouse Snowflake).
Big Data Analytics: Now with AI
The big trend over the last few months in Big Data analytics has been the increasing focus on artificial intelligence (in its various forms and flavors) to help analyze massive amounts of data and derive predictive insights.
The recent resurrection of AI is very much a child of Big Data. The algorithms behind deep learning (the area of AI that gets the most attention these days) were for the most part created decades ago, but it wasn’t until they could be applied to massive amounts of data cheaply and quickly enough that they lived up to their full potential (watch Yann LeCun, pioneer of deep learning and head of AI at Facebook). The relationship between AI and Big Data is so close that some industry experts now think that AI has regretfully “fallen in love with Big Data” (watch Gary Marcus, CEO of Geometric Intelligence).
In turn, AI is now helping Big Data deliver on its promise. The increasing focus on AI/machine learning in analytics corresponds to the logical next step of the evolution of Big Data: now that I have all this data, what insights am I going to extract from it? Of course, that’s where data scientists come in – from the beginning their role has been to implement machine learning and otherwise come up with models to make sense of the data. But increasingly, machine intelligence is assisting data scientists – just by crunching the data, emerging products can extract mathematical formulas (watch Stephen Purpura, founder of Context Relevant ) or automatically build and recommend the data science model that’s most likely to yield the best results (watch Jeremy Achin, CEO of DataRobot). A crop of new AI companies provide products that automate the identification of complex entities such as images (watch Richard Socher, CEO of MetaMind, Matthew Zeiler, CEO of Clarifai, and David Luan, CEO of Dextro) or provide powerful predictive analytics (e.g., our portfolio company HyperScience, currently in stealth).
As unsupervised learning based products spread and improve, it will be interesting to see how their relationship with data scientists evolve – friend or foe? AI is certainly not going to replace data scientists any time soon, but expect to see increasing automation of the simpler tasks that data scientists perform routinely, and big productivity gains as a result.
By all means, AI/machine learning is not the only trend worth noting in Big Data analytics. The general maturation of Big Data BI platforms and their increasingly strong real-time capabilities is an exciting trend (watch Amir Orad, CEO of SiSense and Shant Hovespian, CTO of Arcadia Data )
Big Data Applications: A Real Acceleration
As some of the core infrastructure challenges have been solved, the application layer of Big Data is rapidly building up.
Within the enterprise, a variety of tools has appeared to help business users across many core functions. For example, Big Data applications in sales and marketing help with figuring out which customers are likely to buy, renew or churn, by crunching large amounts of internal and external data, increasingly in real-time. Customer service applications help personalize service; HR applications help figure out how to attract and retain the best employees; etc.
Specialized Big Data applications have been popping up in pretty much any vertical, from healthcare (notably in genomics and drug research) to finance to fashion to law enforcement (watch Scott Crouch, CEO of Mark43 ).
Two trends are worth highlighting.
First, many of those applications are “Big Data Natives” in that they are themselves built on the latest Big Data technologies, and represent an interesting way for customers to leverage Big Data without having to deploy underlying Big Data technologies, since those already come “in a box”, at least for that specific function – for example, our portfolio company ActionIQ is built on Spark (or a variation thereof) , so its customers can leverage the power of Spark in their marketing department without having to actually deploy Spark themselves – no “assembly line” in this case.
Second, AI has made a powerful appearance at the application level as well. For example, in the cat and mouse game that is security, AI is being leveraged extensively to get a leg up on hackers and identify and combat cyberattacks in real time. “Artificially intelligent” hedge funds are starting to appear. A whole AI-driven digital assistant industry has appeared over the last year, automating tasks from scheduling meetings (watch Dennis Mortensen, CEO of x.ai here) to shopping to bringing you just about everything. The degree to which those solutions rely on AI varies greatly, ranging from near 100% automation to “human in the loop” situations where human capabilities are augmented by AI – nonetheless, the trend is clear.
Conclusion
In many ways, we’re still in the early innings of the Big Data phenomenon. While it’s taken a few years, building the infrastructure to store and process massive amounts of data was just the first phase. AI/machine learning is now precipitating a trend towards the emergence of the application layer of Big Data. The combination of Big Data and AI will drive incredible innovation across pretty much every industry. From that perspective, the Big Data opportunity is probably even bigger than people thought.
As Big Data continues to mature, however, the term itself will probably disappear, or become so dated that nobody will use it anymore. It is the ironic fate of successful enabling technologies that they become widespread, then ubiquitous, and eventually invisible.
No comments:
Post a Comment